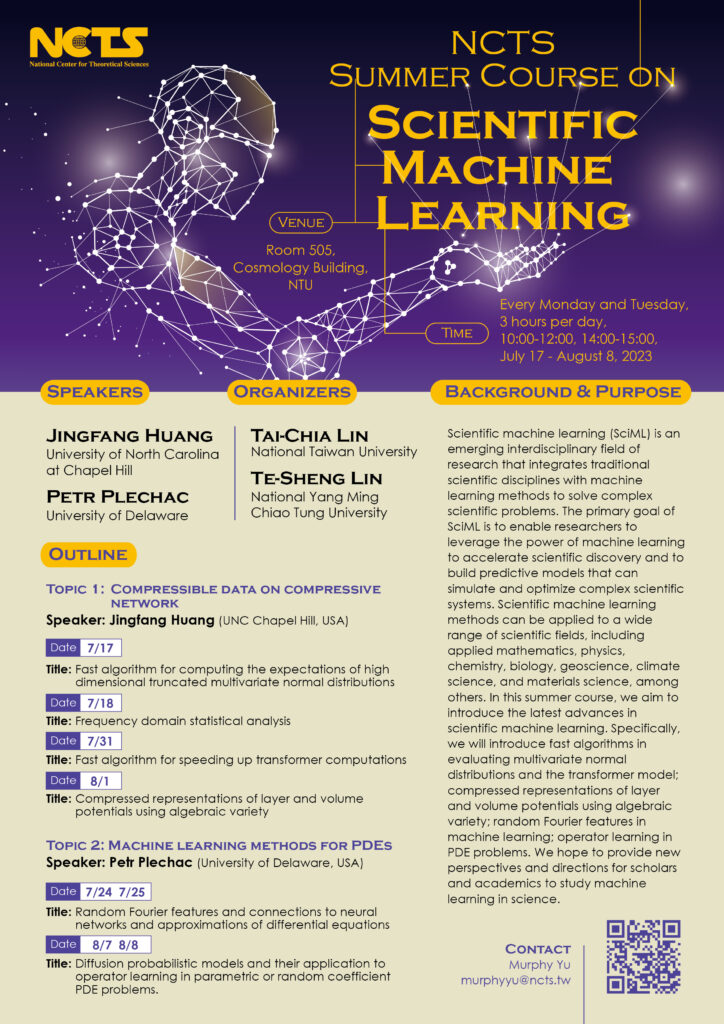
1. Background & Purpose
2. Course Outline
Topic 1: Compressible data on compressive network Speaker: Jingfang Huang (UNC Chapel Hill, USA)
Date: 7/17
Title: Fast algorithm for computing the expectations of high dimensional truncated multivariate normal distributions
Date: 7/18
Title: Frequency domain statistical analysis
Date: 7/31
Title: Fast algorithm for speeding up transformer computations
Date: 8/1
Title: Compressed representations of layer and volume potentials using algebraic variety
Topic 2: Machine learning methods for PDEsSpeaker: Petr Plechac (University of Delaware, USA)
Date: 7/24, 7/25
Title: Random Fourier features and connections to neural networks and approximations of differential equations
Date: 8/7, 8/8
Title: Diffusion probabilistic models and their application to operator learning in parametric or random coefficient PDE problems.Time & Venue
7/17-8/8, Every Monday and Tuesday, 3 hours per day,10:00-12:00, 14:00-15:00, July 17 – August 8, 2023Room 515, Cosmology Building, NTU
Speaker
Jingfang Huang (University of North Carolina at Chapel Hill)
Petr Plechac (University of Delaware)
Registration
Webpage
Contact
Murphy Yu
E-mail:murphyyu@ncts.tw